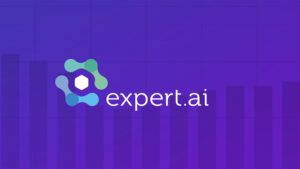
Expert.ai has released a report finding that 77% of businesses with active natural language processing projects plan to increase related spending in the next 12 to 18 months.
“The 2023 Expert NLP Survey Report: Trends driving NLP Investment and Innovation” shows how sought-after NLP technology is becoming for businesses wishing to leverage it to reduce costs, drive growth, and stand out from the competition. Based on research from this survey, Expert.ai reports an increase in use cases ranging from marketing to finance and customer care to sales and attributes this increase to NLP’s ability to interpret the vast quantities of unstructured data that many organizations are collecting.
Of the 77% who predict increased NLP spending, 38% expect to increase their budget by 11% or more. Greater spending is forecast further for companies with more mature NLP models, with 86% of these early adopters anticipating spending increases, 40% of whom are expecting it to rise by 11% or more. Even organizations in the early phases of NLP implementation are expected to increase spending, as 76% of recent adopters said they foresee a greater investment in NLP.
Other notable findings include how 80% of responding organizations have NLP models in production, with 47% in production for less than two years. Data production/governance was named the top business use case for NLP by 47% of respondents, knowledge management and classification came second for 46%, and chatbots ranked third with 46%. The top technical use case for NLP was extraction/named entity recognition for 47% of those surveyed, and intent classification and natural language generation were both named as current or under consideration by 44% of respondents. The financial industry seems to be employing NLP models the most, as 95% of respondents in finance, banking, and insurance have adopted the technology compared to 73% in the technology sector.
Expert.ai says that methodology matters when implementing NLP models, and the survey asked respondents which technology solutions they were using, whether machine learning, symbolic/rules-based approaches, deep learning, graph, or a combination of these. The report notes that over half (52%) use a mixed ML and symbolic/rules-based approach, while 79% employ ML with either DL or graph technology. Compared to this, only 6% use ML alone. The company says that a hybrid AI approach that combines ML and symbolic can deliver highly accurate results in situations where little training data is available, improve explainability and be more predictable than ML-only approaches, and codify domain and process specific knowledge by including SME feedback directly in the rules. This hybrid approach is also more cost effective and energy efficient than computation-heavy ML or neural network strategies, the report states.
“For companies employing NLP solutions for several years, the most compelling arguments for the technology are improvements in efficiency, mitigating risk, and cost reduction. AI has become embedded in their business models, and NLP is allowing them to create new opportunities,” said Marco Varone, Expert.ai founder and CTO. “Still, organizations face challenges aligning with stakeholders over which use cases to prioritize, and in justifying the costs associated with NLP modeling and tools.”
Stakeholder buy-in issues identified by the survey include the financial cost associated with NLP modeling and tools (38%) and agreement on the appropriate use of NLP models (38%). Expert.ai predicts these obstacles will begin to clear as familiarity with NLP increases. The company also asserts that the greatest influencer of stakeholder support for NLP adoption is likely to be the perceived accuracy of results, currently an issue for 37% of organizations, according to survey figures.
Return on investment and how to measure it is another area where some businesses are challenged, as 37% of those surveyed cited building an ROI case for NLP projects as an impediment to adopting the technology. “The traditional calculation of ROI percentage – gains minus cost of investment divided by cost of investment – is made challenging by the need to estimate potential variable operating costs on as-yet undeveloped NLP solutions,” the report states. “One approach to overcome this barrier is using a variety of methods to present the case for NLP to stakeholders, employing differing ROI metrics to track the success of existing models, and so predict the likely returns from new projects.” Expert.ai says its survey data shows that ROI for NLP is being measured based on several factors: time to production (54%), efficiency improvements (53%), and cost reduction (53%).
The report concludes with a few warnings, noting that companies should hedge against upcoming AI regulatory requirements for explainability by adopting responsible AI approaches now. Additionally, given the rapid expansion of NLP technology and use cases, organizations will need a deeper understanding of which technology can deliver the most accurate, repeatable and responsible approaches.
“Without a clear understanding of the varying attributes of alternative technologies, many businesses fail to exploit the full capability of NLP, and risk fumbling the competitive advantage it can deliver,” cautions Varone.
Access the report here.
Related Items:
Taming the ‘White Whale’ of Unstructured Data
Natural Language Processing: The Next Frontier in AI for the Enterprise
John Snow Labs Releases Spark NLP 4.0